Measuring Economic Impact with High-Resolution Satellite Data
Researchers: Gordon Hanson (UC San Diego), Albert Lin (UC San Diego), Jessica Block, Velu Ganapathy, Amit Khandelwal, and Amanda Schochet
Location: India
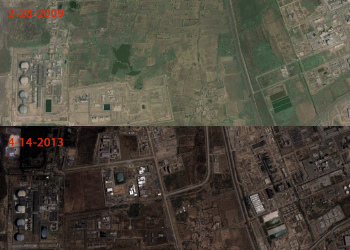
This project uses high-resolution satellite imagery to measure local economic impacts of new business formation. Piloted in Gujarat, India, the results provide new methods for studying the impact of rapid urbanization on socio-economic levels at a global scale.
The project uses satellite images to capture visual changes in economic activity surrounding new industrial plants in the periods before and after plant construction. High-resolution satellite images allow identification of the extent and quality of roads and other transportation infrastructure, the density of buildings and type of materials used in construction, the changes in vegetation type and health, and a host of other features. This, in turn, will facilitate quantifying the environmental impact of vegetation change on issues such as air quality, local ecology and agricultural viability.
This project expands the frontier of remote sensing and computer vision for the analysis of economic development, tapping a new and powerful resource for global observations through satellites. The long-term goal is to make such measurements at scale, across broad regions of the earth using supercomputing.
Ultimately, researchers foresee public, private, and/or government access to the increasingly massive volumes of remote sensing data will provide a profound resource for the observation and investigation of global change. Yet understanding the power of each pixel and the methods required in extracting insight out of those pixels at scale is challenging. This pilot study begins to explore this in the context of economic development in India, where the benefit of remote sensing is that it provides quantitative output in an ecosystem lacking consistent reporting.